How the Steam Engine Can Help Us Make Sense of AI
Plus, why ChatGPT was a turning point for the markets, and legal and regulatory challenges around AI.
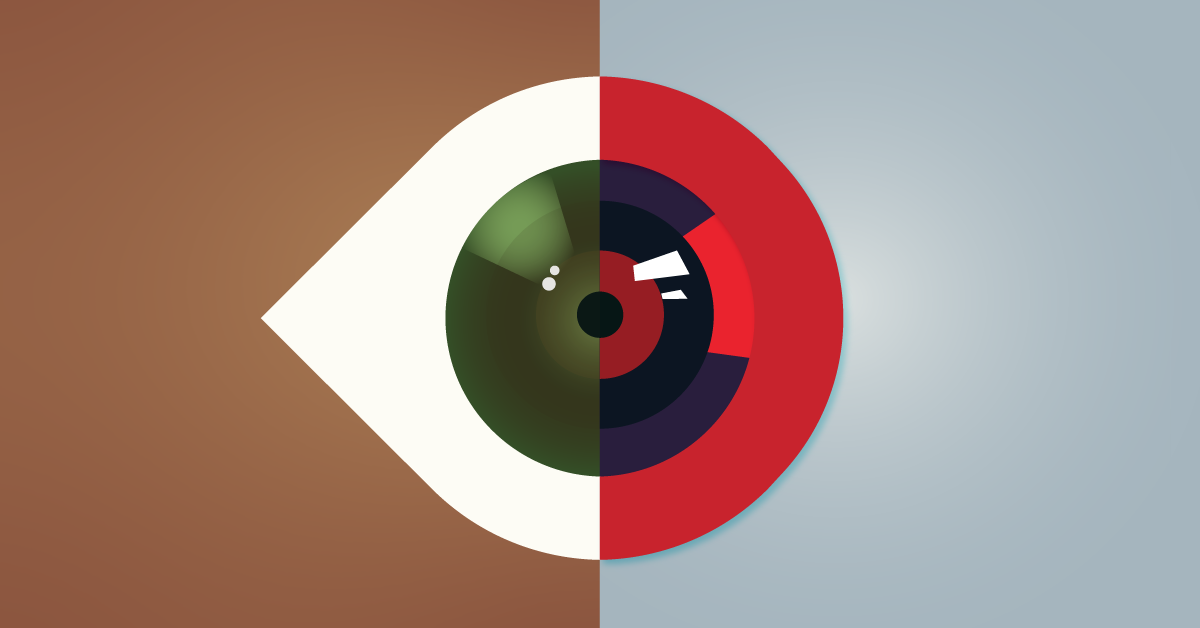
On this episode of The Long View, Ankur Crawford, senior vice president and portfolio manager at Fred Alger Management, breaks down how ChatGPT and generative artificial intelligence has affected the markets, what innovation lies ahead for AI, and the dominance of growth stocks in the market.
Here are a few excerpts from Crawford’s conversation with Morningstar’s Christine Benz and Dan Lefkovitz.
Why ChatGPT Was a Turning Point for the Markets
Dan Lefkovitz: We wanted to zoom out a little bit and ask you about AI. You mentioned that you think we’re in the early stages of a long-term transformation. For many of us, ChatGPT, when it launched in late 2022 was a real a-ha moment in terms of gen AI and what it’s capable of. It certainly seems to be a turning point for markets. Were you as surprised as the rest of us by ChatGPT? Did you see it as a significant milestone?
Ankur Crawford: Yes, I was as surprised about ChatGPT. But maybe for different reasons than the market was surprised by it. So, given that our philosophy is to look for change, we’ve been looking at AI, and I would argue at that point it was machine learning since the 2015 and 2016, and we started talking and thinking about digital transformation. We’ve done many videos and thought pieces about AI. AI is nothing to fear in 2017 and ‘18. In part because we knew that AI was going to be a structural imperative once it came onto the scene. The reason that ChatGPT was really interesting and exciting is because ChatGPT isn’t just like LLM like Chatbot. What it does and what all of these large language models do is they democratize compute. And one of the reasons that AI and INTERNET OF THINGS, and all of these innovations, didn’t take hold over the last 10 years has been because the compute costs were so expensive that it was almost too expensive to really implement these kinds of technologies. However, when ChatGPT burst onto the scene and you got these large language models that have basically ingested all of the data that they can get their hands on, it changes the equation because effectively what you’ve done is what I said before, you’ve democratized compute. And as you democratize compute and that compute itself continues to get cheaper and cheaper because the innovations that the chip manufacturers are currently producing, you end up getting products and businesses that end up being able to use and be more productive and it doesn’t cost them an arm and a leg to deploy the technology. So, the productivity gain is greater than the cost that you have to incur to deploy the product or to deploy AI. And I think that’s what’s really exciting about gen AI. It has made the cost of this compute accessible for all. And that is the key, the golden key, for why a decade from now our world will look so incredibly different than it looks today because the cost structures are the ROI on deploying AI is going to be vastly positive, especially over the next two to five years.
How the Steam Engine Can Help Us Make Sense of AI
Christine Benz: We’ve heard lots of historical parallels invoked to help make sense of AI, the internet, railroads, electricity, even the discovery of fire. So, do you find any of these historical parallels to be useful?
Crawford: I do. Actually, I find one in particular very useful, which is the steam engine. And I came about this simply because I was helping my, at the time, 13-year-old study the industrial revolution in Europe and what had happened over the course of really 100 years. And it’s really interesting because when the steam engine burst onto the scene—it actually didn’t burst; it was a very slow ramp. And at the time, I believe that Spain was the major economic force in the world. And India, they were exporting a lot of cloth and spices to the West. However, what’s interesting is that the UK was the first to adopt the steam engine and in part because at the time, the cost of labor in UK was significantly higher than in India and China. So, for them, the ROI of deploying a steam engine into a factory and making their own cloth was quite positive. And what it did, it allowed them to start manufacturing cloth. They started buying raw materials from India. And they started to manufacture cloth on their own and effectively disrupted the entire Indian economy that was based on the creation of fabric.
Which gave UK enough both money and might to then become an imperial power over the next 200 years. So, that was one really interesting aspect of the industrial revolution with the steam engine that I found very interesting and has some parallels to today. The other aspect is clearly, what it did to the labor force. England was largely an agrarian society. And they had to transition to a more industrial society where a lot of the farmers then had to figure out how to work in factories and things like labor laws, child labor laws had to get put in place. And so there was a great disruption to the labor force that took about a generation for the country to adjust to this new kind of labor need. And as you think through what’s about to happen with AI, I feel like this might happen at a pace that’s faster than a generation. I think it might happen in half a generation because the adoption rates for this and the productivity gains are going to be significant. So, I think that there are interesting parallels to what we’re going to have to do in order to train and retrain our labor force as we move from a non-AI world to a more AI-based world.
Skepticism Around AI
Lefkovitz: Earlier you referenced some of the skepticism that’s out there about AI. Some of the skepticism surrounds the cost of the technology, the environmental impact. There have been questions about how much value it can add, like what huge problem it’s solving. How do you how do you think about some of those questions?
Crawford: Oh, gosh, there’s a lot there. And what I’m going to tell you now, I can tell you is it might be wrong. Because at this point, we have to simply think about the implications of a new world that is using a highly productive workforce. And what the implications are for the environment or for labor, and what I will tell you—and I feel highly confident of—is that the productivity gains will be significant. And let me give you an example of a company that we own called Cadence. Cadence Design Systems is a company that basically makes software that allows engineers to make chips, and you can’t make a semiconductor without using a piece of software like Cadence. Cadence is basically a duopoly-type market, incredible management team, incredible products. And they have seen that in their own usage of AI, they’ve taken their product cycle for their hardware that they make, and they design from six years to three years. So, they cut the product design timeline by half. It’s hard to quantify what that’s worth to them, but it’s significant. Their own engineers are anywhere from 20% to 30% more efficient as they’re designing chips. A typical chip design engineer, a fully loaded cost, maybe from $300,000 to $500,000. If an engineer is 30% more productive, there is a dollar value that’s associated with that productivity.
That’s very tangible. So, I think there are many examples of productivity gains or benefits of using AI. I think as the cost of that compute declines over time, the number of different productivity use cases that open up just become more and more significant from a consumer-based AI app that I expect Apple will introduce eventually or from enterprise-based apps that will eventually show productivity. Everyone complains about Copilot, for example. And they’re like, well, Microsoft’s Copilot, it’s only 10% productive. I will remind everyone that, ChatGPT burst onto the scene, as you had mentioned Dan, only two years ago, approximately. And so, we are in the very, very early innings of this adventure. And you can’t expect the whole world to change inside of two years. But the trajectory of where we’re going is really exciting because when software begins to write software, innovation becomes exponential. It is no longer linear and limited by humans. And I think that’s the big takeaway that when software begins to write software, innovation is exponential.
Potential Legal and Regulatory Challenges With AI
Benz: We wanted to ask about potential legal and regulatory challenges. We’re told that you know something about intellectual property. You hold some patents. And it’s been pointed out that AI models are trained on data and IP without necessarily getting permission for it. You earlier pointed out all of the data that ChatGPT ingests. Do you see that as a risk?
Crawford: I think, Christine, that it is something that we have to watch. As any technology matures over time there are puts and takes and just like we had to put in child labor laws back in the industrial revolution when children were being put to work. In the same way, we have to put guardrails around any technology that bursts onto the scene like this. So, is there going to be some IP issues around using data? There will be, and we’ll have to solve them as we progress. I think these are all issues. The regulatory environment—I’m a firm believer that if you regulate today, it will A) stop innovation. But also, the environment is changing so fast. So, if you say, “OK well, I’m going to regulate a model that has 500 billion parameters.” OK, well the models are moving faster than we can actually put regulatory rules in. And so, I fundamentally believe if we regulate too fast on this gen AI, we’ll probably regulate to the wrong metric because the world is moving too fast, and we haven’t yet matured to a point where we know where we sit. And so, what are we regulating to? It’s a tricky period because you want to protect things like IP, but our government can’t be too heavy-handed either.
The author or authors do not own shares in any securities mentioned in this article. Find out about Morningstar’s editorial policies.